This is an Eval Central archive copy, find the original at camman-evaluation.com.
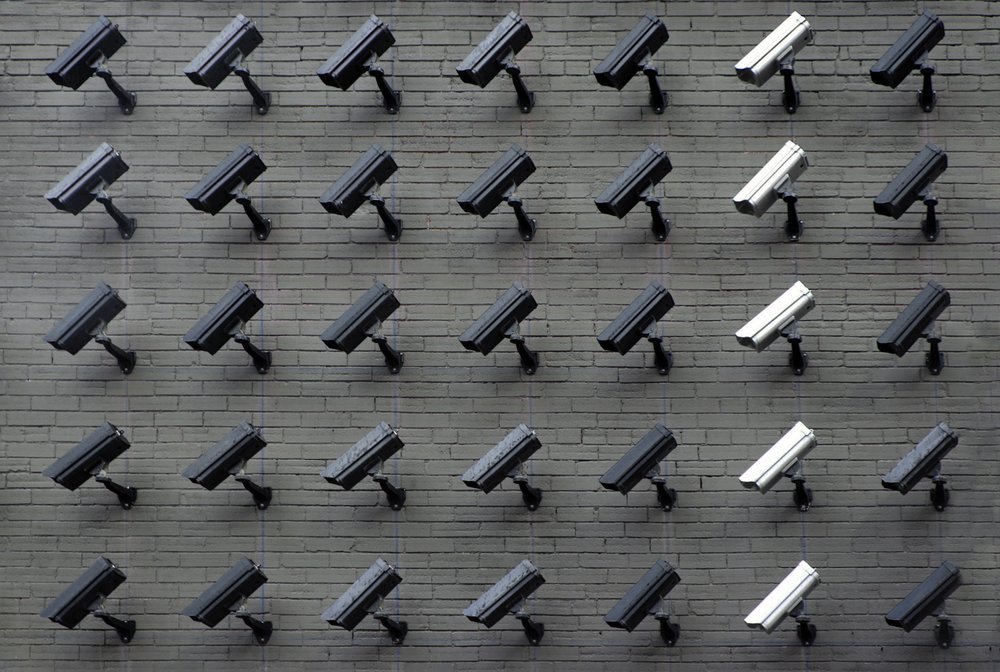
Photo by Lianhao Qu on Unsplash
“Can we even get that data?”
That question, or some version of it, is usually one of the first, if not the first, question I hear when planning or discussing a new evaluation project. People want to know if it’s possible to collect data on a particular outcome or from a particular group. There’s often an undertone of, “I bet we can’t,” in the question too.
From the outside, I think evaluation must look a lot like survey + interviews = report. Those are the parts of the process that most people actually get to interact with after all. (Which is too bad, because they are also the least impactful parts to experience in terms of process use.) So I can understand why questions about data collection (and reporting deliverables) often dominate early conversations I have.
But data collection is not the hardest part of evaluation. My answer to, “Can we even get that data?”, is “Yep, probably.” In some way, in some form, we can get data on that or from that group of people. But there are so many more essential questions to be asked first. Do we need that data? What are we going to use it for? Do we have a plan for how to use it? Do we have the people, resources, and processes in place to make sure it’s going to get used?
Because here is the thing. If you have gasoline, you generally also want—at minimum—a gas-powered vehicle, a capable driver, and a destination in mind or at least a general direction to start in, otherwise you’ve got a lot of something you can’t use very effectively. Same goes for data. And while you want to factor stopping for gas into your road trip plans, it’s probably not where you start the planning process.
A flaw in that analogy though is that it doesn’t reflect just how much of a problem it can when we focus on data collection to the detriment of data use. When we collect data we don’t need and can’t use, or *do* need but fail to use well, the consequences can range from wasteful to, in the most extreme circumstances, deadly.
Julia Coffman wrote up one of the most compelling examples of this in her article, Between the Devil and the Deep Blue Sea: The Consequences of Small Failures in Learning. In it, she explores a fatal shipwreck caused by what should have been an avoidable collision with a hurricane, had it not been for the persistent misuse of data (in this case meteorological data about the course of the incoming storm). There was enough of the necessary data to avoid the collision as well as people highly qualified to interpret it on the ship, but fatal decisions were still made. Julia explains the cognitive and interpersonal biases as well as situational factors that may have contributed to the misinterpretation and misapplication of data, though we can’t know for certain as the main decision-maker—the captain—died along with his crew.
Just having data doesn’t mean we will use it well, and having too much data or the wrong kind of data can be misleading as well as a waste of resources. As this article from the Stanford Social Innovation Review points out, with technological advances it’s getting easier and easier (and cheaper) to collect, store, and analyze data. But a tool like a dashboard only produces higher-level data of your raw data, it can’t tell you what it all means or how to use it effectively. That requires human-level interpretation and application, which isn’t getting any faster or cheaper or easier and isn’t helped by a flood of irrelevant information without a meaningful practice for making sense or use of it.
The latest example I’ve found of how easily and chronically we end up in these bad habits with data is a is New Yorker review of various books on the history of spies and intelligence agencies, which sums up the main takeaway as, “The history of espionage is a lesson in paradox: the better your intelligence, the dumber your conduct; the more you know, the less you anticipate.” Yep, that’s right—there’s a long and storied tradition of intelligence-gathering being self-defeating and counter-productive.
Espionage and evaluation differ many ways , but fundamentally we’re still talking about data-gathering to inform decision-making and we’re in the territory of human fallibility, so many of the problems surfaced in the article sounded awfully familiar. For example, “Not for the first or the last time, the point of spying—to know what the other side is likely to do—had been swallowed up by the activity of spying,” reminds me of this statement from another article on over-measurement practices, “Micro-measuring what we have done seems to be more important than what we actually do.” Process subverting purpose!
The problem of volume and data overload comes through in this comment, “if you have any secret information at all, you often have too much to know what matters”. And the issue of focusing on the wrong kind of data, “The two agencies were so busy spying on each other, it almost seems, that they forgot to spy on each other’s government. Knowing what the K.G.B. was doing wasn’t the same thing as knowing where the Soviet state was heading, and the rise of Mikhail Gorbachev and the fall of the Soviet Union came as a complete surprise to the C.I.A.” There’s also a repeated pattern of accurate, useful intelligence being suppressed or ignored because of “… confusion, political rivalry, mutual bureaucratic suspicions, intergovernmental competition, and fear of the press (as well as leaks to the press), all seasoned with dashes of sexual jealousy and adulterous intrigue.” (Okay, those last two probably come up less often in evaluation, but the rest are commonplace!)
The role of trust and transparency in the misuse of intelligence fascinated me too. “The universal law of unintended consequences rules with a special ferocity in espionage and covert action, because pervasive secrecy rules out the small, mid-course corrections that are possible in normal social pursuits. When you have to prevent people from finding out what you’re doing and telling you if you’re doing it well, you don’t find out that you didn’t do it well until you realize just how badly you did it.”
Maybe this is less relevant to the use of evaluation findings since there technically we’re not required to operate under a shroud of secrecy the same way spies are. But don’t we end up doing so much of the time anyway? Evaluation is political, and a lot of it happens quietly, in-house, with little-to-no publicly available documentation, and minimal participation by most people affected by the process in anything other than providing data and maybe having access to the final report. While participatory approaches to evaluation seem to be gaining in usage, I’m not sure they’re anywhere near being the norm. There can be a real culture of fear around data and how it might be used to reflect badly on organization or program that prevents, or at least seriously limits, actual useful discussion and application of it.
I won’t minimize the political nature of data and how it can be weaponized, but I’ll offer that blanket secrecy is not necessarily the most effective way to manage that risk, especially with the cost to utility. This is where the value of having a clear evaluation strategy—a direction and purpose behind why the evaluation is being conducted and how it will be translated into use—can benefit again. It ensures that data are collected with purpose and intention and can be interpreted and applied in light of that purpose, with a clear explanation of why that data, that interpretation, and that use. The more robust the evaluative reasoning, the harder anyone else is going to have to work to offer a counter. And, bonus, you end up with a better evaluation either way.