This is an Eval Central archive copy, find the original at camman-evaluation.com.
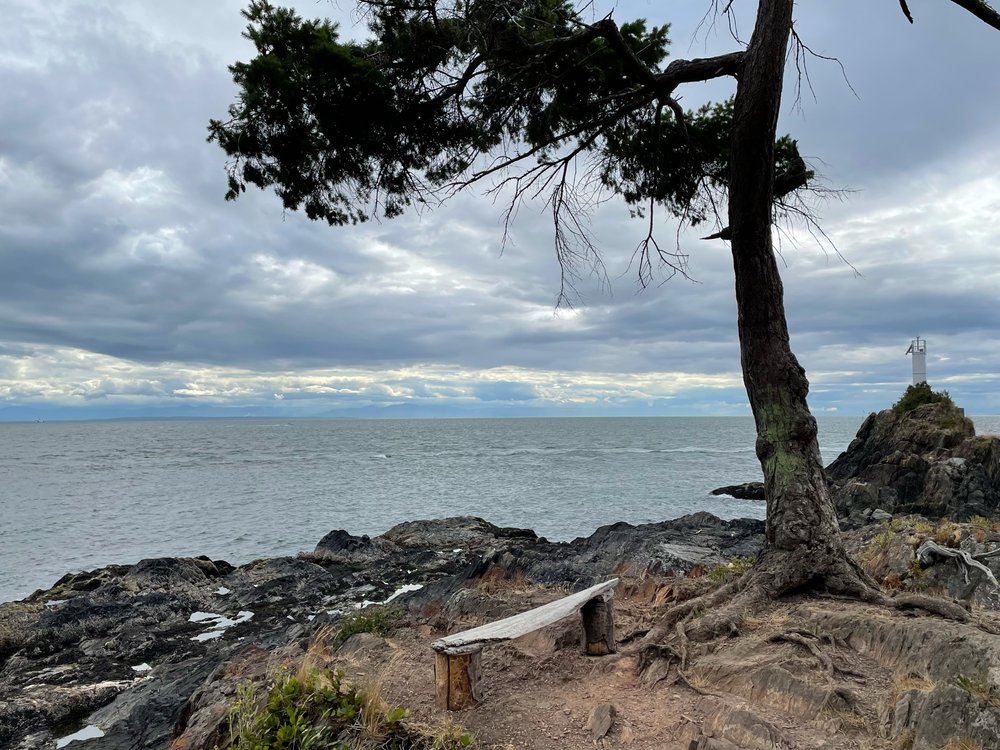
The view from Cape Roger Curtis over the Salish Sea on Nex̱wlélex̱wm/Bowen Island.
My friend Chris Corrigan recently wrote a great blog post on weather and complexity, riffing off a statement from a retiring weather forecaster to talk about how to navigate complexity. One of my favourite COVID-era hobbies was tracking weather patterns with Chris and our friend Amanda. As systems swept in and out over the coast, we would announce in our group text the moment when rain reached our respective locations, from Nex̱wlélex̱wm/Bowen Island to East Van to New Westminister. Chris always has a fascinating app or person he follows on Twitter with cool maps and data about what is actually happening and the three of us got quite nerdy about it. (I’ll never forget on the first night of the heat dome, when he showed me a heat map visualizing that column of hot, red air going straight up to the highest levels of the atmosphere, sitting on top of us with nowhere to go. Terrifying.)
So when I need a simple way to illustrate how data alone is not the answer to our evaluation challenges, I often find myself using weather forecasting. It’s something that we’re all familiar with in a general sense, but often don’t have a full appreciation of what’s actually going on behind the scenes. It also speaks to the very practical circumstances of our day-to-day lives, including the fact that we live in a world of increasingly dangerous weather events and climate change.
Weather is also something we can collect fairly concrete data about. These are physical phenomena that we can measure directly with reasonable precision and reliability. We can then subject this data to some pretty sophisticated mathematical modelling and make decently accurate predictions about what will happen at least briefly into the future. The meteorologist that Chris’s post quotes foresees a future into which our statistical clairvoyance can still improve by leaps and bounds with new technological breakthroughs.
Yet data collection and even data analysis are only one part of what we need. A sentence that stood out to me from the quote at the start of that post, “The atmosphere is a nonlinear system, meaning our ability to forecast it is extremely sensitive to knowing the exact condition of every breath of air”, really gets at the scope of the challenge. Highly-connected, interdependent complex systems are limitless and irreproducible in models. We cannot capture every single small element that might affect what happens next. Not to mention, because these systems are non-linear, where inputs and outputs are not proportionate (i.e., ‘the butterfly effect’), even the tiniest unmeasured element, like a breath of air, might end up being an integral part of a complex emergence. Add to that the logistical cost and complexity of collecting, managing, and analyzing high volumes of data, which is is limited not just by the human capacity to do so but literally the physical computer processing power available. And this again is relatively concrete data that lends itself to this kind of statistical modelling (versus the rigamarole of developing abstract proxy indicators of non-quantitative concepts, like “wellbeing” or “motivation” or “knowledge”, which do not have universally-agreed upon definitions much less direct modes of measurement).
On top of all of that, even with all of the data we can get and the physical ability to analyze and make sense of it, and the historical and theoretical knowledge to build reasonably accurate predictive models about it, not only is that prediction still always going to be inherently limited and fallible (which does not detract from the work of the forecasters), but having all of that in hand still only at best offers us somewhat more information with which we must still ultimately make a decision about what to do. And even that information will be highly context-specific and have a fairly short shelf-life of relevance. Alongside getting a heads-up that my area is at risk of an extreme weather event within the next six hours, I need to have my own contingencies in place and hope I live in a place with a well-resourced and well-designed emergency response plan and the capacity and political wherewithal to carry it out. That also
No shade to the meteorologists, of course! Their job is to make sure the information is timely, accessible, and reliable, and that’s important. But data will never tell us what to do or how to do it or make sure that it’s acted on well—that’s on us.
And you may think that evaluation isn’t about forecasting, it’s about accountability and learning and looking to the past to describe and report on what happened and what has been accomplished. But in practical terms, many of us approach evaluation with the idea (rightly or wrongly) that what has happened in the past will happen again the same way in the future. When we ask, “Does the program work?” (a question situated in a generic present tense), the logic of evaluation is to look at how it has (and hasn’t) worked already and extrapolate into a present (and presumably future) tense based on that. When program sponsors decide to fund or support a particular initiative, they are doing so with an eye to the future and what they hope or believe or want to see happen, usually with a lot less concrete data to go on than what the weather forecasters are working with. We look to the past the same way we use a mirror to look at the back of our heads—to see what we can’t see.
If you think the solution to the uncertainty and guesswork of this process is “data-driven decision-making”, I refer you again to the sentence, “our ability to forecast it is extremely sensitive to knowing the exact condition of every breath of air”, and ask, who will build and fund this data infrastructure and make sure it is available to and appropriate for everyone (so as not to design inequity into the system from the start)? Even the meteorologist notes, “we crudely sample the atmosphere directly with instruments that aren’t precise and numerous enough, and make even more approximations with remote sensing like satellites”. These are multi-billion investments over decades that are still underfunded and less developed than they could be.
As Chris makes the point in his post, these models do not give us much insight into hyper-local conditions, which are greatly impacted by the geological specificities of our exact contexts. Saying, “This program works. It’s a great intervention.” does not account for the particularities of how a program might play out at another time or in another place. And the answer to that is not ‘more data’ and ‘better models’, but better situational awareness and attention to the context of the present moment, more acknowledgement of our agency and responsibility in the decisions we make about our social interventions, and looking to the data available to us with a critical eye for useful insights rather than definite answers.