This is an Eval Central archive copy, find the original at evalacademy.com.
As evaluators, we are rarely organizational decision-makers; it is our job to provide those decision-makers with actionable insights. In this article I highlight how you can translate data into meaningful findings, or insights, so you can support decision-makers to drive action within their organizations.
Asking the right questions
The process of deriving actionable insights from data starts with asking the right evaluation questions. What do your clients need to answer to tell their stories? Evaluation questions are the starting point to any analysis and the answers are the end point. As the anchors of your analysis, it is crucial to dedicate time with your clients to iron out these evaluation questions; they will provide needed context to all results garnered from the analysis.
If you are struggling with writing evaluation questions, we have previously written about how to write evaluation questions (with sample evaluation questions). Refer to these articles for more details on establishing effective evaluation questions.
Start with the data that you have
Make life easier on yourself: start with the data that you have. Data collection takes time. Rather than expending a bunch of resources on data collection, evaluate whether current data sources are sufficient to answer the evaluation questions.
However, there will be instances when the data required to address the evaluation questions do not exist. In this case, you may need to develop data collection tools (e.g., surveys, interviews) to collect relevant data. Keep things simple and focus on the evaluation questions. Anchoring the data and analysis in your evaluation questions maintains focus, limiting the ability for a project’s scope to creep beyond what was originally agreed upon.
Which data collection tool you select will depend on the evaluation question and your evaluation design or approach. However, surveys are usually a quick and cost-effective method for collecting data. For example, your evaluation question may ask: “To what extent do patients have a positive experience with primary care programs and services?” To answer this question, we could design a patient survey. The survey could include questions on satisfaction with specific programs and services or overall satisfaction with primary care. The survey should ask questions that will directly address the overall evaluation question. Fewer direct questions are recommended over many tangential or unrelated questions.
While this is a simplified example, a survey is not limited to answering a single evaluation question. Survey tools can be designed to capture data for one or many evaluation questions. The key is to make sure all questions align with your evaluation questions; this will focus the survey and capture data relevant to the overall goal of your evaluation.
“Garbage in, garbage out”
The results of any data analysis are only as good as the data themselves. If data quality is not ensured, the results of your analysis will be suspect and likely invalid. It is critical that data are scrutinized prior to analysis to establish confidence in the results and insights drawn from the analysis. Therefore, prior to analysis, data quality needs to be evaluated on:
-
Completeness – are the data sufficiently complete to address your evaluation questions?
-
Accuracy – do the data correctly reflect the data being collected?
-
Consistency – do data reflect the same information within and across data sources?
-
Validity – do the data align with pre-determined conditions/ formats?
-
Uniqueness – are data represented once within a given data set?
-
Timeliness – are data up to date to adequately address your evaluation questions?
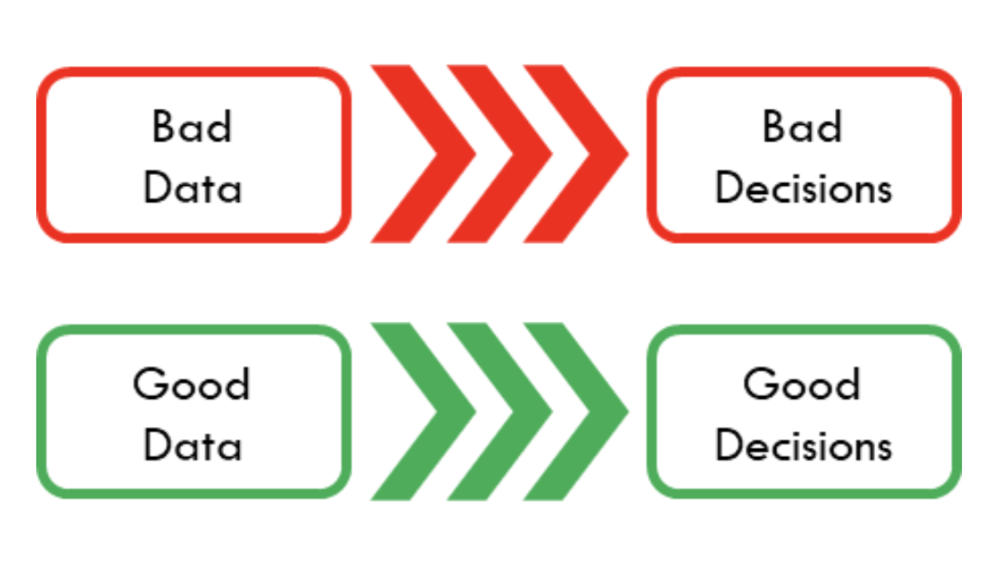
Likely, data will not meet all dimensions of data quality right away. Some dimensions of data quality can be fixed with simple data cleaning (e.g., correcting minor typos and formatting dates). Other times data points may be excluded from the analysis. However, it is crucial that the data meet all dimensions of data quality prior to analysis to ensure accurate results.
Data to information
Spreadsheets, regardless of their size and complexity, only store data. That is, a spreadsheet does not provide any meaningful information until the data are structured and organized in a meaningful way. Analysis takes the data building blocks and structures them into something useful (i.e., information). This information will, again, be tied back to the evaluation questions outlined prior to the analysis.
Information may be summarized as numbers (e.g., proportions, tables) or images (e.g., charts, infographics). How information is structured and presented are dependent on the context of the evaluation questions asked. The key is to provide information that is simple and easy to interpret.
Sample “information”:
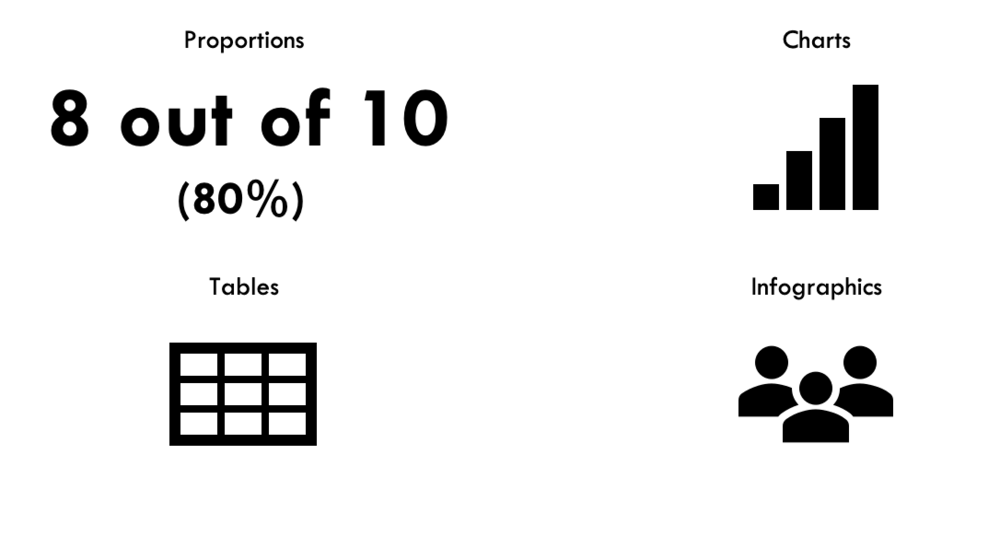
Information should focus on meanings. What do the data illustrate? How does the information connect to the evaluation questions? This is accomplished by focusing the information. That is, focus on one major point per piece of information. By narrowing the focus, you are better able to communicate that information with decision-makers.
Actionable insights
Now that data have been converted into information, it is time to take that information and transform it into actionable insight. Actionable insights come from taking the information gleaned from an analysis and getting at the “so what?”
Getting at the “so what?” is not always easy. But there are a few approaches to move insight to actionable insight, including:
-
Segmenting (or grouping) the results
-
Using data visualizations to support the results
-
Comparing to benchmarks (e.g., time series, norms)
-
Adding additional context
Segmentation
Segmenting data into discernable groups can help get at the “so what?” Segments, such as demographics, split the results of the analysis into comparable groups. Which segments you investigate are dependent on the evaluation questions asked.
Looking within an organization? Segment by department to derive insight into potential departmental differences.
Looking at financial literacy outcomes? Segment by age or gender to derive insight into potential learner differences.
Segmenting the information derived from your analysis may help identify patterns in the results. Patterns may identify important differences between segments that will allow for the client to better develop an action plan.
Data visualizations
Data visualizations, such as charts and infographics, do not inherently provide actionable insights. However, they can provide additional support for the key findings of an analysis. Effective data visualizations can highlight key messages within the data and help identify areas for action.
Take this result: 80% of patients were satisfied with their last visit.
On its own, we only have one piece of the story. Did the remaining 20% of patient feel neutral about their last visit? Or were they very dissatisfied? For this example, providing a chart with the statement can provide additional context. Knowing that 20% of patients were dissatisfied with their last visit is likely to spur more action than if the patients had neutral feelings about their last visit.
Benchmarks
Further insights may be gleaned from benchmarks. These may be internal (e.g., comparing between time points) or external (e.g., comparing to standard norm). Using benchmarks can get at the “so what?” and provide valuable context to the results of an analysis.
Looking at the previous example, exploring the results over time could provide additional context. For example, if 100% of patients were satisfied with their last visit in 2020 and 80% of patients were satisfied with their last visit in 2021, we can immediately identify a decrease in patient satisfaction. However, if 60% of patients were satisfied with their last visit in 2020, we would likely see a different response from the client. Providing results with the additional context of a benchmark has the potential to turn information into an actionable insight.
Additional context
As evaluators, it is not necessarily within the scope of our role to expand beyond what is provided in the data. Sometimes the data do not fully lend themselves to actionable insights. These cases require additional context beyond the data.
At this point, it is time to hand the results off to your client. Your client will have a better understanding of internal operations, processes, or biases within their organization. Their expertise can provide additional context not apparent from the data alone and the client can come up with their own conclusions based on the results.
The roadmap for transforming data into actionable insights starts and ends with asking the right evaluation questions. These questions guide the entire analysis process, moving data to information and information to actionable insight. The goal is derive meaning from data and answer the “so what?” questions to help organizations target areas for action.
Sign up for our newsletter
We’ll let you know about our new content, and curate the best new evaluation resources from around the web!
We respect your privacy.
Thank you!
Sources:
How to Write Good Evaluation Questions
Scope Creep: When to Indulge It, and When to Avoid It
7 Tips for Better Data Visualizations
How (and Whether) to Write Recommendations
What Is Data Quality and Why Is It Important?